In this stream of work we develop AI-based methods to evaluate decision-makers, such as to inform compensation and optimal assignments of experts to tasks. We develop machine learning techniques as well as algorithms for cost-effective use of online labor markets for machine learning.
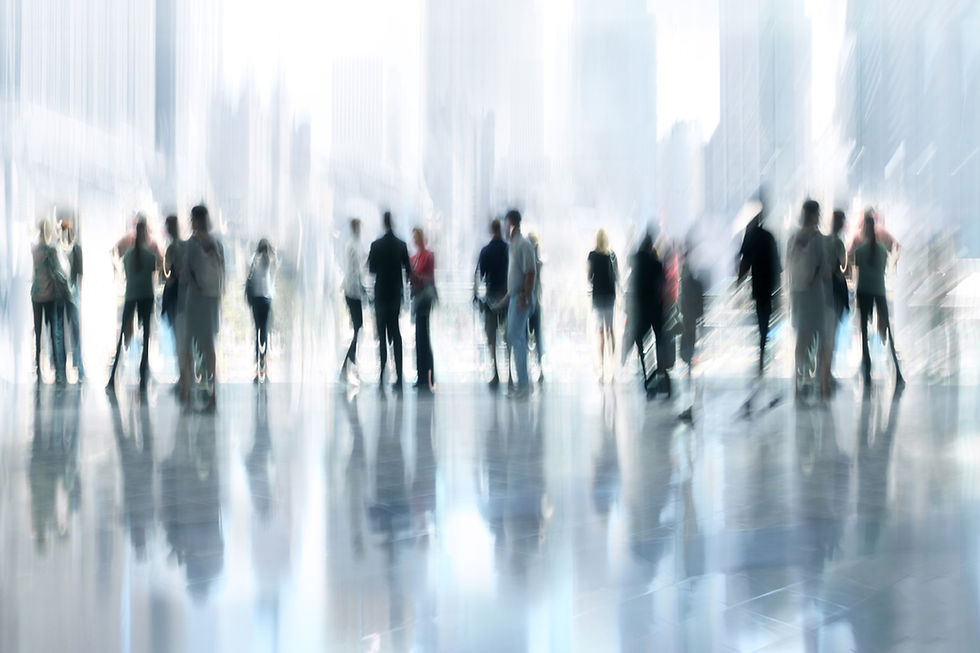
Scalable & Costless Ranking of Experts
The capacity to rank expert workers by their decision quality is a key managerial task of substantial significance to business operations. However, when no ground truth information is available on experts’ decisions, the evaluation of expert workers typically requires enlisting peer‐experts, and this form of evaluation is prohibitively costly in many important settings. In this work, we develop a data‐driven approach for producing effective rankings based on the decision quality of expert workers; our approach leverages historical data on past decisions, which are commonly available in organizational information systems. Specifically, we first formulate a new business data science problem: Ranking Expert decision makers’ unobserved decision Quality (REQ) using only historical decision data and excluding evaluation by peer experts. The REQ problem is challenging because the correct decisions in our settings are unknown (unobserved) and because some of the information used by decision makers might not be available for retrospective evaluation. To address the REQ problem, we develop a machine‐learning–based approach and analytically and empirically explore conditions under which our approach is advantageous. Our empirical results over diverse settings and datasets show that our method yields robust performance: Its rankings of expert workers are consistently either superior or at least comparable to those obtained by the best alternative approach. Accordingly, our method constitutes a de facto benchmark for future research on the REQ problem.
More For Less: Adaptive labeling payments in online labor markets for Machine Learning
In many predictive tasks where human intelligence is needed to label training instances, online crowdsourcing markets have emerged as promising platforms for large-scale, cost-effective labeling. However, these platforms also introduce significant challenges that must be addressed in order for these opportunities to materialize. In particular, it has been shown that different trade-offs between payment offered to labelers and the quality of labeling arise at different times, possibly as a result of different market conditions and even the nature of the tasks themselves. Because the underlying mechanism giving rise to different trade-offs is not well understood, for any given labeling task and at any given time, it is not known which labeling payments to offer in the market so as to produce accurate models cost-effectively. Importantly, because in these markets the acquired labels are not always correct, determining the expected effect of labels acquired at any given payment on the improvement in model performance is particularly challenging. Effective and reliable methods for dealing with these challenges are essential to enable a growing reliance on these promising and increasingly popular labor markets for large-scale labeling. In this paper, we first present this new problem of Adaptive Labeling Payment (ALP): how to learn and sequentially adapt the payment offered to crowd labelers before they undertake a labeling task, so as to produce a given predictive performance cost-effectively. We then develop an ALP approach and discuss the key challenges it aims to address so as to yield consistently good performance. We evaluate our approach extensively over a wide variety of market conditions. Our results demonstrate that the ALP method we propose yields significant cost savings and robust performance across different settings. As such, our ALP approach can be used as a benchmark for future mechanisms to determine cost-effective selection of labeling payments.
How to Bring Transparency in Experts Markets?
We propose a problem and develop a machine-learning-based method to enhance transparency in important human decision making markets, where little transparency is available today. We focus on the decision making accuracy of expert, such as of physicians who make non trivial (e.g., diagnostic) decisions. Decision accuracy is a fundamental aspect of experts judgment quality, and thus limited transparency about experts’ decision accuracies undermines effective management of experts resources as well as consumers’ choices. In the health care domain, for example, poor transparency has lead to consumers reliance of uninformative alternatives to identify suitable experts, which research has shown is poorly correlated with objective medical performance measures.
We consider settings where decision makers are costly, and make arbitrarily complex decisions for which ground truth is rarely available, including after the decisions are made (e.g., because ground truth is costly to acquire). Such settings arise in key fields, including the medical domain, where for some diagnoses, ground truth is rarely acquired or recorded ex post. Because experts are costly, continuous acquisition of peer- committee evaluations from multiple of experts to establish the ground truth on an expert’s past decisions is prohibitively costly as well.
Comments